Digital simulation and digital twins: optimizing time, cost and business processes
In the age of technological innovation, companies in every industry face increasing pressure to reduce costs and time while maintaining high quality standards. The adoption of digital simulation systems and digital twins is revolutionizing design, manufacturing, maintenance and logistics processes . These advanced tools make it possible to anticipate the results of physical testing, improving efficiency and lowering the costs associated with iterative cycles at different stages of operation.
Digital simulation: a bridge between theory and practice
Digital simulation is the process of replicating a physical system or phenomenon using mathematical models and computer algorithms. In practice, a digital simulation creates a virtual model of a process, system or environment, allowing different scenarios to be tested without the risks and costs associated with using real machinery. not yet developed or built.
Digital simulation is used in various industries to test products, systems, processes . Applied at the design stage, it enables the creation of 2D or 3D digital models through computer-aided design (CAD) software, which can also include mathematical algorithms to represent parts of a process or product. The main goal is to reduce prototyping costs by replacing physical prototypes with fully virtual models.
These models allow highly realistic simulations: if a product has defects, the digital model data can be modified directly without having to create a physical prototype. An additional advantage is the ability to test various operational scenarios to optimize quality and productivity, identifying the optimal configuration before moving to production.
Traditionally, simulations have been used to evaluate system designs and verify their ability to meet operational objectives. For example, in the automotive industry, it is possible to virtually test the structural strength of a prototype vehicle in a crash test, identify weaknesses and make corrections quickly and inexpensively.
Digital twins: creating virtual twins
Digital twins further expand the potential of simulation. A digital twin is more than just a model that integrates real-world objects into the digital world. It is a dynamic virtual representation of an existing physical object, system or process that is continuously updated with real data. The digital twin communicates bidirectionally and continuously: it measures changes in the physical system via sensors and constantly shares predictions with the physical system to make real-time decisions. Companies such as IBM, Siemens and GE are already adopting digital twins.
Digital twins enable enterprises to optimize performance, predict future problems and make real-time adjustments. Powered by advanced Industry 4.0 technologies such as Internet of Things (IoT) sensors, artificial intelligence (AI), machine learning (ML), cloud computing and data analytics, digital twins are constantly connected to real-time data sources. Sensors embedded in physical counterparts send live data to the virtual model, ensuring synchronization with reality.
These digital twins not only simulate, but also monitor and optimize performance throughout the product life cycle. For example, an industrial plant equipped with digital twins can predict machine failures, plan preventive maintenance and improve overall operational efficiency. In addition, they make it possible to simulate material wear and tear over time, thanks to knowledge of physical laws, to optimize the timing of maintenance or replacement of components.
Digital modeling with digital twins, therefore, is key to reducing the risk of costly plant downtime and maximizing productivity. With digital twins, companies optimize processes dynamically and continuously, adapting quickly to changing market needs. This approach makes it possible to simulate not only products, but entire industrial plants or even complex systems such as the human body, opening up new possibilities in medical and engineering fields.
Comparison of digital simulation and digital twins
DIGITAL SIMULATION |
DIGITAL TWINS. |
|
Purpose |
Instrumental or predictive testing and analysis of physical systems and phenomena, advanced training, risk management, process optimization, and strategic planning |
Real-time monitoring, optimization, and predictive maintenance of systems and processes |
Data and interaction |
They use predefined data sets and may not incorporate real-time updates. Interaction takes place before the simulation, setting static and one-way data and parameters in order to test different conditions and scenarios and observe the results |
Data flow between the physical system and the digital twin is continuous, bidirectional, and real-time to continuously update the virtual model. The digital twin allows interaction with the model to dynamically test different scenarios |
Investment |
It is contained because of its easy configurability, absence of real-time data, and seamless integration with the physical system |
It is conspicuous because, to effectively represent the physical system, sensor data must be integrated with real-time analytics and AI |
Life cycle |
In general, the use of digital simulation occurs for a short period of the life cycle in order to provide the desired results in specific scenarios |
Digital twins maintain an ongoing relationship with their physical counterparts throughout the life cycle. Use is for medium to long periods, and the twin evolves hand in hand with the replicated physical system |
Model creation |
Simulation models can vary in complexity, from simple statistical models to complex and detailed representations |
Digital twins require multilayer models that can replicate real-world counterparts |
Applications of digital simulation and digital twins
Starting from the design phases and continuing into the industrialization and production phases, and applications of digital simulation and digital twins in business processes offer tangible benefits in terms of optimization of time, cost, quality and sustainability.
Depending on the needs, they can be applied individually or in an integrated mode. Let us look together at the applications in the two approaches for some key areas.
Applications of simulation
Mechanics
Through simulations of wear, friction and stress, mechanical components, such as gears or motors, are optimized. This reduces maintenance costs and improves the performance of industrial machinery.
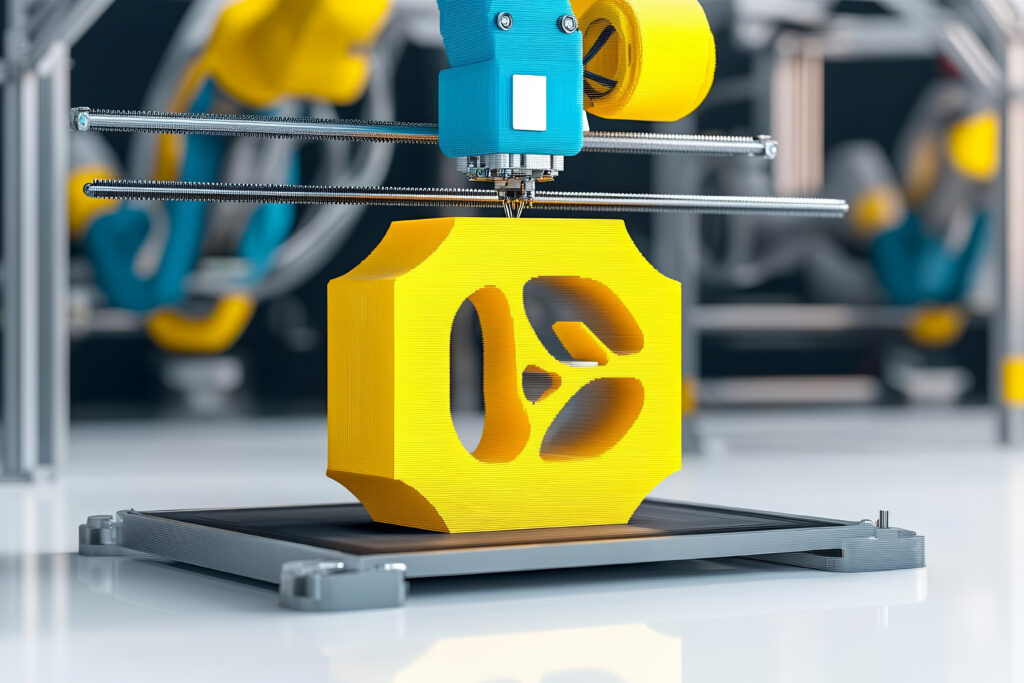
Plastic
By modeling the injection molding process, defects in manufactured parts are reduced, material use is optimized, and the quality of finished products is improved.
Applications of digital twins
Aerospace
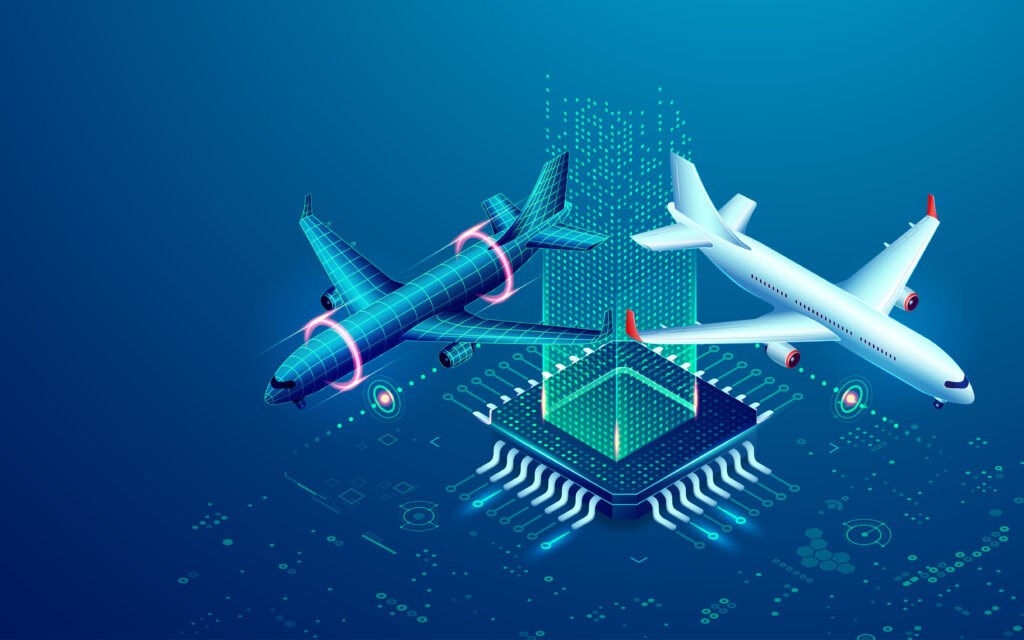
Airbus uses digital twins across its entire fleet of aircraft to monitor flight data in real time, maintain optimal conditions and anticipate problems before they occur. Through virtual replicas of its aircraft, Airbus can simulate different scenarios, evaluate performance, and make informed decisions. Boeing’s integration of digital twin technology into aircraft design and production is an example of its potential. In this case, a digital twin was used to simulate aircraft parts and analyze how different materials will behave throughout the aircraft’s life cycle. Through these calculations, Boeing was able to achieve a 40 percent improvement in the quality of some designed parts.
Automotive
Tesla is at the forefront of using digital twins to design, test, and optimize its electric vehicles before they even get to the production line. Using simulations with digital twins, Tesla has improved product quality, reduced development time, and streamlined production processes.
Mixed applications of digital simulation and digital twins
Manufacturing
An automobile manufacturer wishing to improve assembly line efficiency can use digital twins to create a replica of the current production process. By integrating them with simulation, changes such as the reorganization of assembly stations or the introduction of robotic arms can be tested. Thus the impact of these changes on production, product quality and worker safety can be predicted, ensuring that the changes are in line with the desired results.
Energy
With simulation of wind and photovoltaic plants, production is optimized and maintenance costs are reduced. General Electric (GE) leverages digital twin technology to predict failures and reduce downtime of wind turbines. By constantly analyzing data from various turbine components, GE is able to optimize its processes, increase energy production, and extend the life of its equipment.